Research in Department of Electrical and Computer Engineering, Computational Intelligence Laboratory.
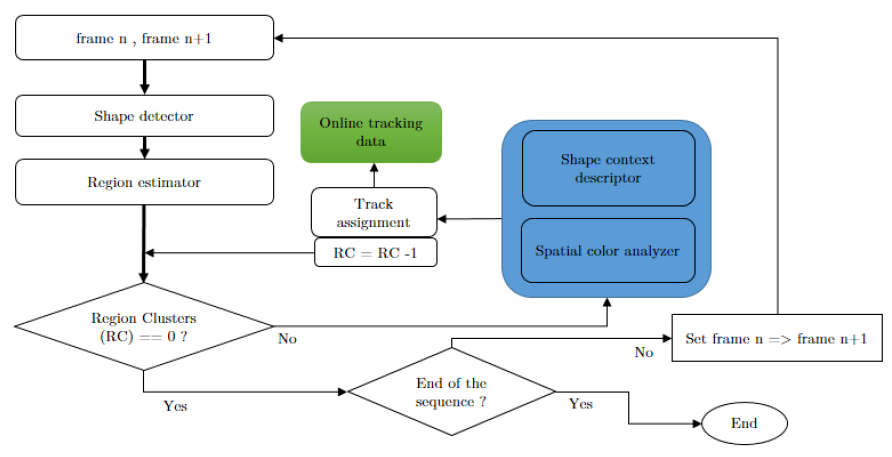
Near sets that includes descriptive proximity spaces and proximal conjugacy as well as a broad range of applications of proximal signals (see, e.g., J.F. Peters, T. Vergili, Fixed point property of amenable planar vortexes. Appl. Gen. Topol. 22 (2021), no. 2, 385-397, under publications, and M. Shangol Haider, Pedestrian Pose Estimation Using Near Set Theory, M.Sc. Thesis, 2021, under dissertations,
Machine learning that includes video frame foreground detection and classification (see, e.g., M.S. Haider, J.F. Peters, Temporal proximiities: self-similar temporally close shapes, Chaos, Solitons and Fractals 151 (2021), 111237, https://doi.org/10.1016/j.chaos.2021.111237 and
Digital image processing that includes voxel lumens flux self-similarities, discrete as well as continuous analysis of image cell complexes (see, e.g., M. Borkowski, Digital image processing in measurement of ice thickness on power lines, M.Sc. thesis, 2002).
Computational topology that includes applications in the graphics derived from transforms of cell complex signals found in the absorption and reflected light from curved surfaces such as river ice, plants, road and building surfaces (see, e.g., under dissertations and Arjuna Philipu Hewa Don, Video Sharpness Barcodes with Bessel Functions and Quaternions, Ph.D. thesis, 2022 and A. Don , J.F. Peters, S. Ramanna, A. Tozzi: Quaternionic views of rs-FMRi hierarchical brain activation regions. Discovery of multilevel brain activation region intensities in rs-FRMI video frames, Chaos, Solitons and Fractals 152 (2021), 111351, Paper.
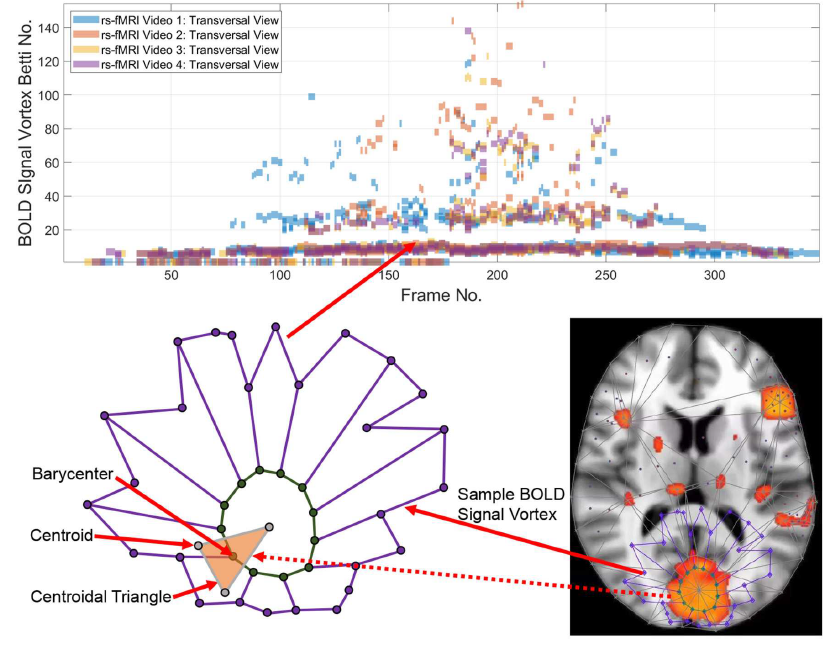
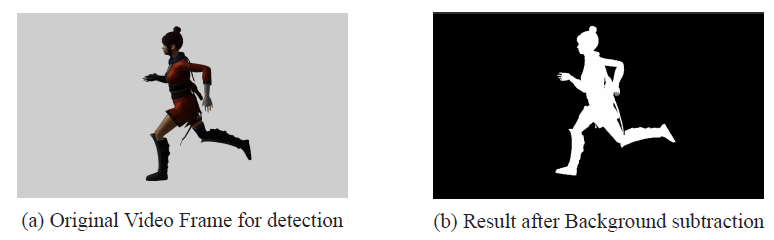
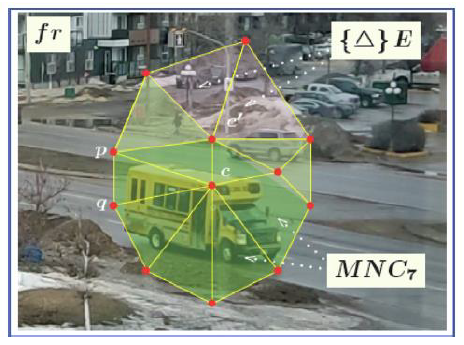
The CI laboratory engages in fundamental as well as applied research in Computational Intelligence. In parallel to ongoing research projects, the objective of the Laboratory is to provide the community with fully updated information on CI. It is an indispensable source of current information encompassing internal reports, relevant publications and providing with a number of links to selected sites worldwide.
Last updated on October, 2022 02:41