December
2024
Transcriptomics
Oshlak A, Robinson MD, Young MD (2010) From RNA-seq reads to
differential expression results. BMC Genome Biology 11:220. http://genomebiology.com/2010/11/12/220.
Trapnell, C et al. (2012) Differential gene expression and
transcript expression analysis of RNA-seq experiments with TopHat
and Cufflinks. Nature Protocols 7:562-578.
doi:10.1038/nprot.2012.016.
Cresko Lab RNA-seqlopedia http://rnaseq.uoregon.edu/
Thiru, P RNA-seq: Methods and Applications. http://jura.wi.mit.edu/bio/education/hot_topics/RNAseq/RNA_Seq.pdf
A.
Overview
1.
Types of data
2. What we are trying to learn
B.
Microarrays have largely been superseded by RNAseq
C.
Experimental considerations for RNAseq
1. Sources of experimental variation
2. Experimental design
3. RNA
4. Sequencing technologies
D. Transcriptomic Data pipelines
1. de-novo
assemblies vs. assembly by read mapping
2. Normalization
3. Which genes show a "significant" difference
between treatments?
4. Differential expression
A.
Overview
1)
Types of data
Transcriptomic studies
tend to generate two different types of data. Studies in which two
or more conditions are compared at a time generate discrete state
data. Often it is critical to follow the expression of a gene over
time after a treatment. In timecourse experiments, the expression
of each gene in response to two or more treatments is measured
over time. For example, in the timecourse at right, the solid blue
and red dashed curves might represent the expression levels for a
gene in response to two different drugs.
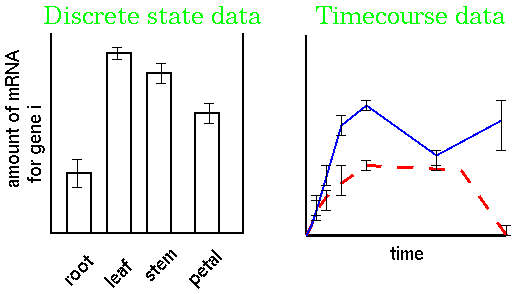
There
is a whole family of problems in normalization of data and
controlling for components of experimental variation.
To put things into
perspective, if the experiment was repeated 4 times, the
timecourse above represents
2 treatments x 6 times x 4 replicates =
48 RNA populations to be sequenced
to generate the data.
Although the data for each
replicate are averaged, there is often a great deal of
variation in the results, which can potentially negate any
meaning. Therefore, extraordinary measures must be taken to
minimize experimental variation at each step in the procedure, to
minimize the overall variation.
2.
What are we trying to learn from transcriptomics?
The
primary goal of transcriptomic experiments is to generate
expression information for every gene in the array, under some set
of condittions. Expression may be studied in
- different tissues
- different developmental stages
- different genotypes
- different treatments
- different times after a treatment.
The kind
of results that are sought in transcriptomic experiments can be
illustrated as follows:
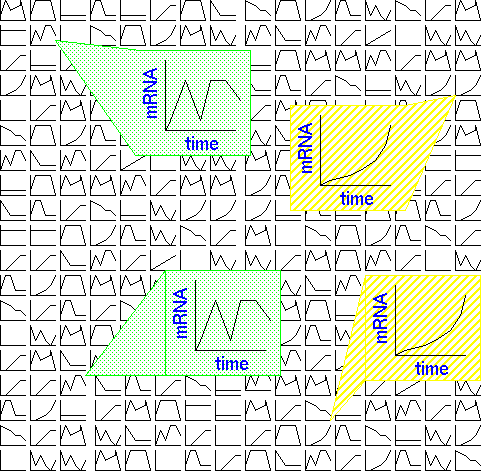
In the example,
timecourse data are generated for each transcript in an RNA
population. The raw data consists of a series of expression
curves for timecourses, or histograms where other types of
treatments are being compared. The goal is usually to find which
groups of genes have the most similar expression patterns. In
the example, two genes (hatched background) show a gradual
induction over the period of the timecourse. Two other genes
(shaded background) show a biphasic response with two distinct
periods of strong expression.
Key questions:
- Which genes are expressed
differentially, between condition A and
condition B?
- How can genes be grouped
according to similarities in expression patterns?
|
B.
Microarrays have largely been superseded by RNAseq
RNA-seq has become the
method of choice for transcriptomics.because RNA-seq directly
counts cDNA copies of mRNAs, it has fewer sources of
experimental variance than microarrays. Because RNA-seq has now become
cost-competitive with microarrays, and the costs of sequencing
keep going down, RNA-seq is rapidly replacing microarrays.
Comparison of
sources of experimental error
|
Microarrays
|
RNA-seq
|
requires previously sequenced and annotated
genome
|
NA
|
error in quantitation of RNA can affect ratios
of expression compared between two treatments
|
NA
|
cDNA synthesis/labeling
|
NA
|
quality of array
|
NA
|
hybridization
|
NA
|
washing
|
NA
|
measurement of signal
|
NA
|
NA - not
applicable
|
|